We combine data science, machine learning, and dynamical modeling to generate infectious disease intelligence and to develop a predictive strategy to confront growing threats to global health. Our work is responsive to current global health events, such as the SARS-CoV-2 pandemic and outbreaks of Ebola, Zika, and Nipah viruses, and applies ecological and biological first principles to extract actionable, data-driven predictions that inform management and outbreak preparedness.
Machine Learning to Predict Zoonotic Disease Risk
Multiple ongoing projects address questions such as: Why do most emerging human diseases originate from wildlife? Where and when is this most likely to occur? What distinguishes the small fraction of species that carry and transmit these zoonoses to humans? Intrinsic organismal characteristics (e.g., life history, ecological, physiological traits) recapitulate a long evolutionary history that may signal species' capacity to be future reservoirs, vectors, or pathogenic agents of zoonotic disease. By examining particular species groups (mammal orders), vector groups (ticks, mosquitoes), and pathogen and parasite types, this work aims to understand the biological underpinnings of zoonotic diseases, and connect these patterns to make predictions about disease risks on a rapidly changing planet.
ACTIVE PROJECTS: NIH CREATE-NEO | NSF-NIFA EEID | NSF US-UK EEID | ClimateIQ | NSF Integration Institute (Verena)
PAST PROJECTS: NSF PIPP Phase I | DARPA PREEMPT | NSF EEID Global Zoonoses
Return to Top
Macroecology of Zoonotic Disease
Zoonotic diseases are distributed across space, time, and species. Our lab focuses on answering outstanding 'who, what, where' questions about zoonotic diseases. Where are zoonotic reservoirs currently distributed, and where are their hotspots and coldspots? What particular species pose the greatest spillover risk to humans? When do zoonotic disease threats grow and when do they diminish?
Many of our projects focus on two overarching goals: 1) to inform global health preparedness and improve ecologically comprehensive disease management, and 2) to draw out mechanistic hypotheses about the outbreak and emergence process from empirical data, especially 3) the mechanisms through which macroecological patterns emerge from multiple interlinked biological scales.
Example projects include spatiotemporal risk modeling to understand the emergence ecology of Ebola and related filoviruses; identifying wild reservoirs to prevent long-term endemicity of Zika virus in the Americas; predicting novel tick vectors and understanding why some ticks are better than others at transmitting zoonoses; an NSF EEID funded project to understand global trait patterns of zoonotic diseases across mammal groups and the consequences of these host traits for disease dynamics in host populations; a multinational DARPA funded project to understand drivers of henipavirus shedding and to develop therapeutic interventions for spillover prevention in bats.
ACTIVE PROJECTS: NIH CREATE-NEO | NSF Integration Institute (Verena)
PAST PROJECTS: NSF EEID Global Zoononses | DARPA PREEMPT
Mechanistic Links Across Systems and Scales
We aim to uncover the mechanisms that drive zoonotic disease risk across all biological scales, from molecular and cellular processes to ecosystem dynamics. These mechanisms will be impacted by the systems in which they are embedded, for instance, agricultural systems, rapidly urbanizing systems, or due to changes in climate. These aims require understanding how interactions at different scales contribute to the broader, macroecological patterns of zoonotic disease emergence and transmission. Our research seeks to connect the dots between fine-scale biological mechanisms and large-scale ecological processes, enabling us to better predict and mitigate disease outbreaks in a holistic, integrated manner.
ACTIVE PROJECTS: EEID SARS-2 | ClimateIQ
PAST PROJECTS: DARPA PREEMPT
Return to Top
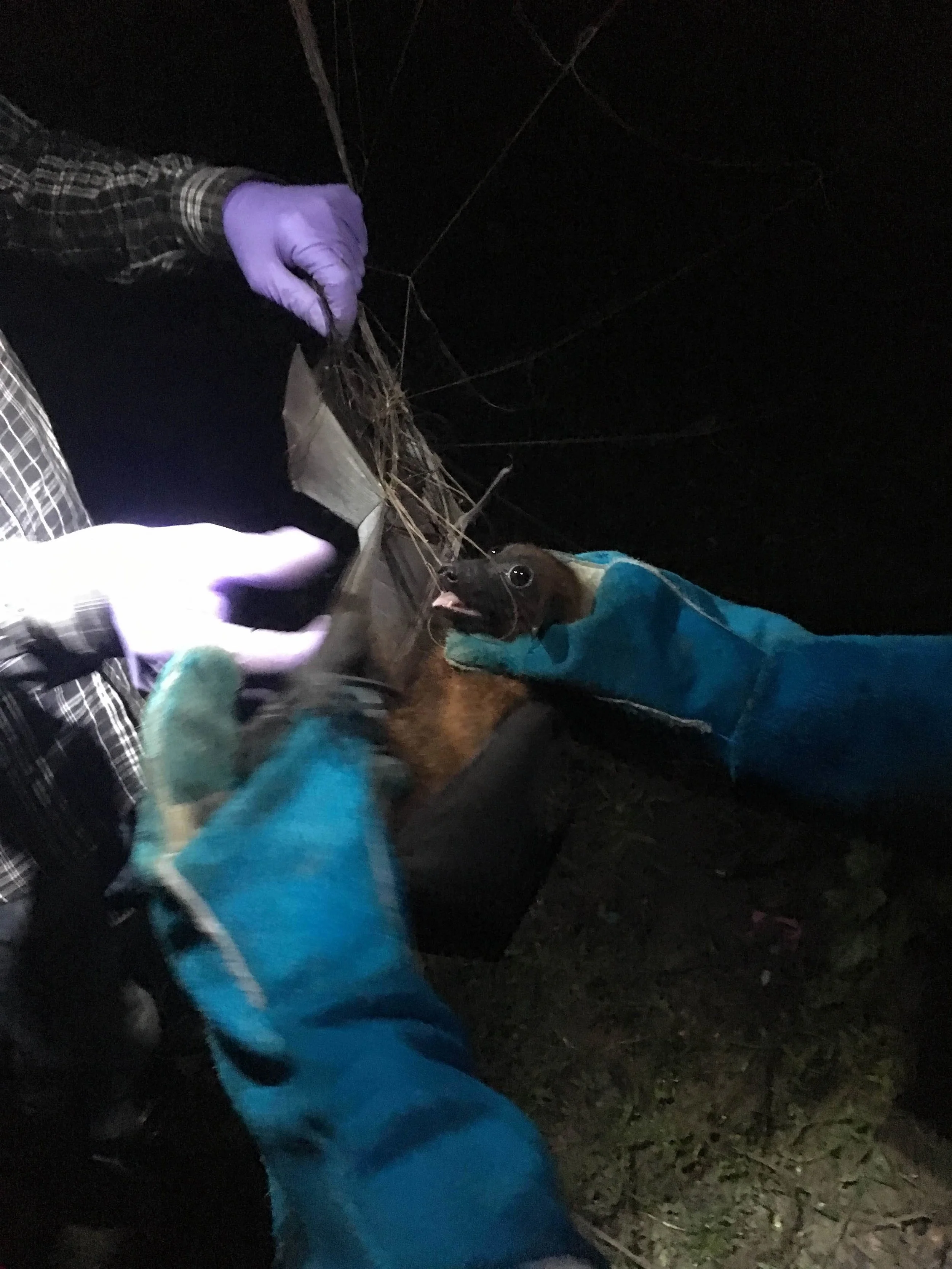

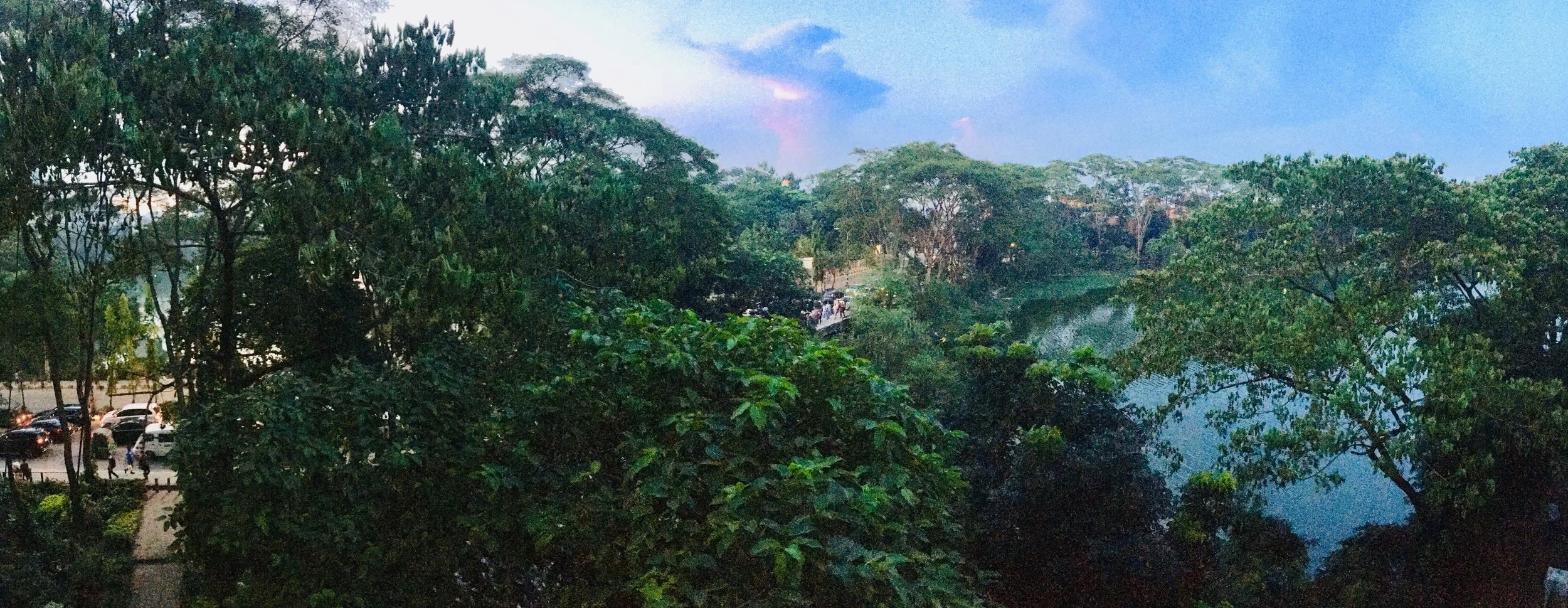
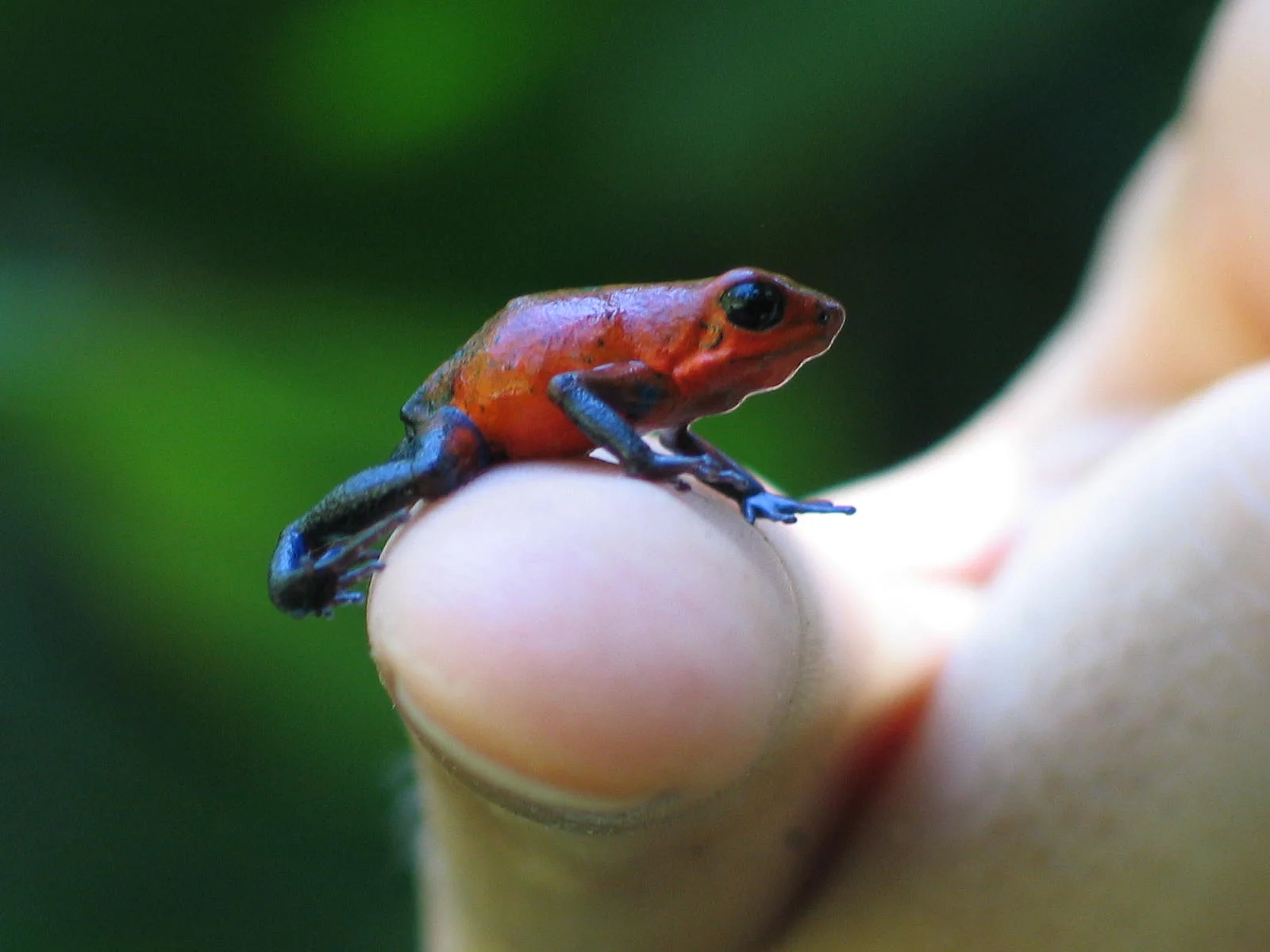





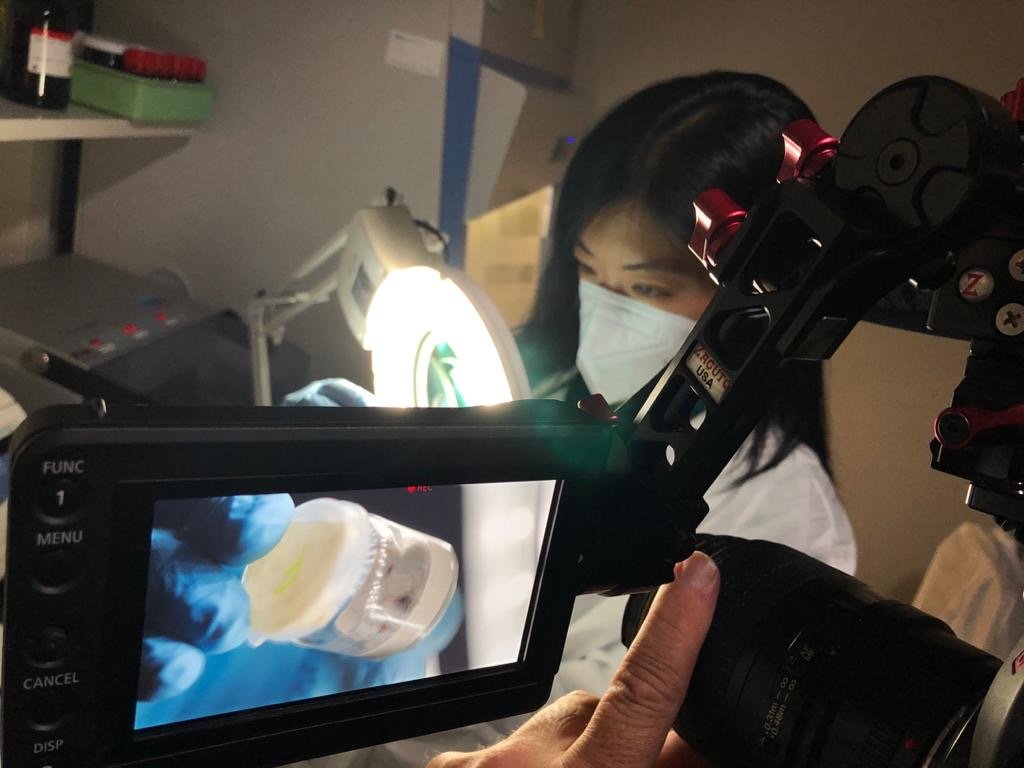



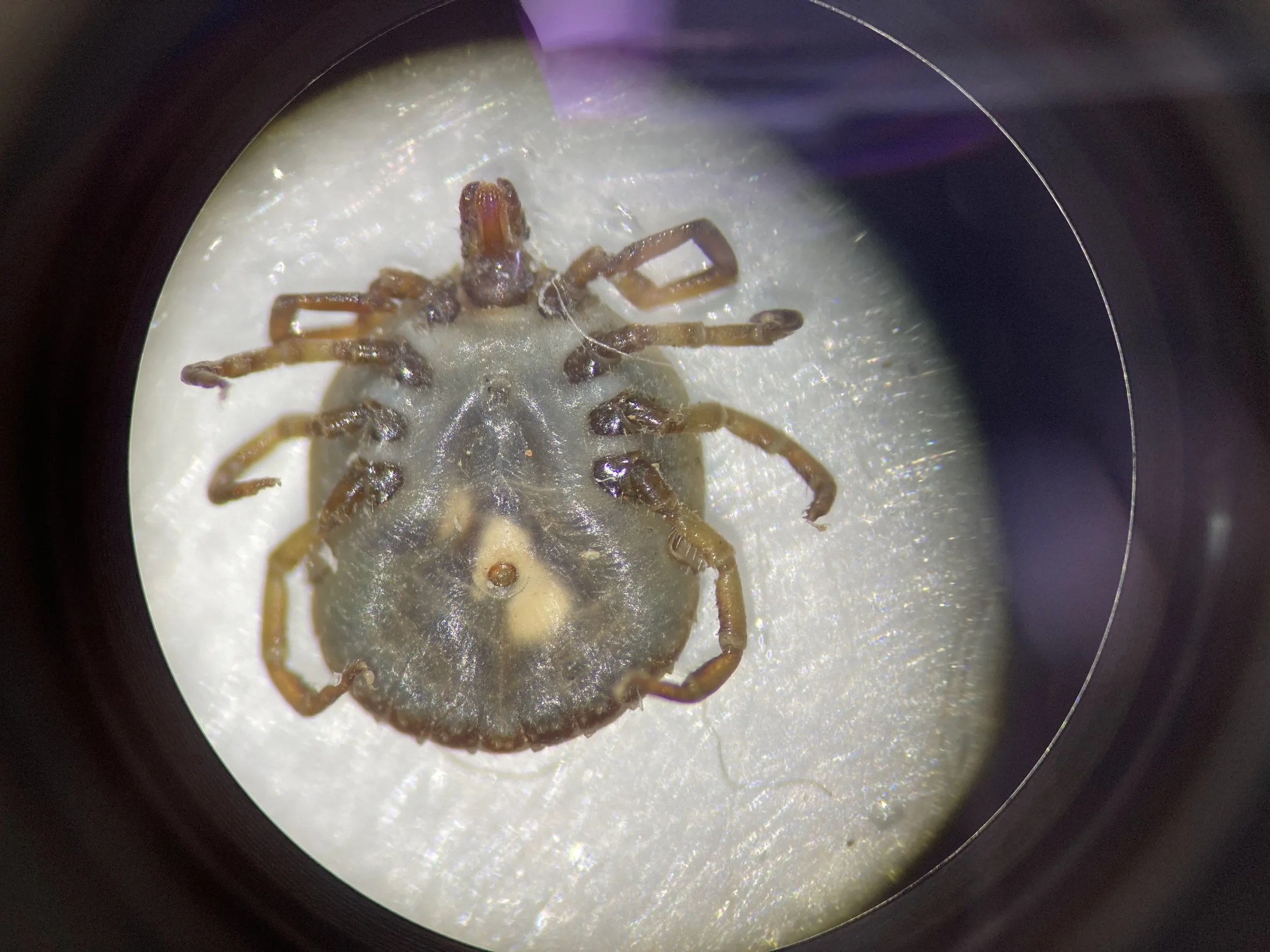




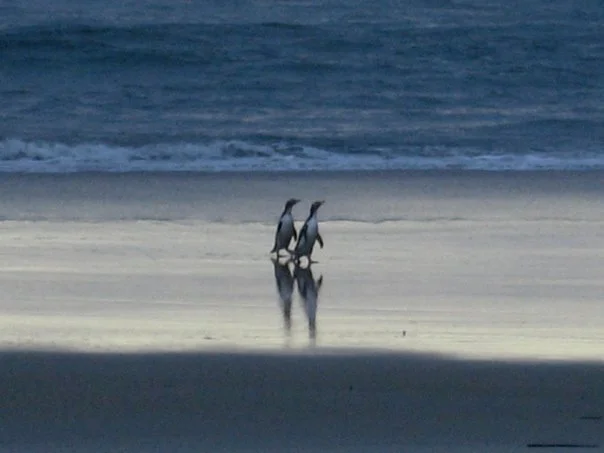

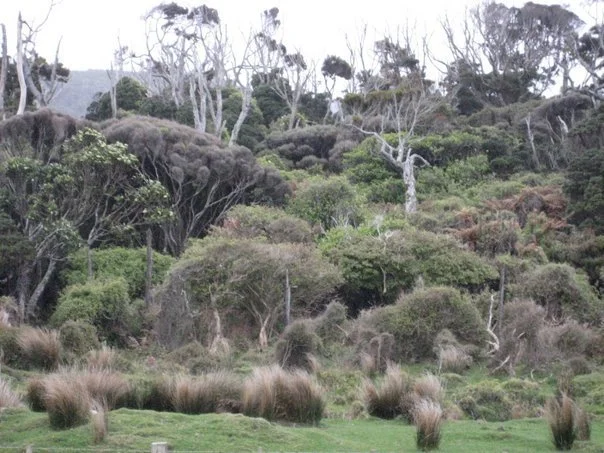